Weather predictions are challenging under many aspects.
Nowadays, we have numerical weather prediction models (NWP), which are complex software able to solve mathematical equations by exploiting high-performance computers.
The NWP equations describe how some atmospheric variables, like pressure and temperature, change in time and space.
By looking at the evolution of those variables in the atmosphere, NWP models predict weather conditions, which are characterized by a specific combination of the considered variables.
To have realistic and reliable forecasts, the NWP machinery must be fed by observations of these variables.
Temperature, pressure, wind speed and direction and other observations are collected from weather stations, with dedicated sensors, and properly transferred to meteorological agencies, which use them for predictions.
A GNSS (Global Navigation Satellite System) station returns observations of the time spent by signals emitted from a constellation of satellites to reach the station itself. The interaction with water vapour decreases the signal propagation velocity, therefore increasing this time, or in – other words – producing a delay.
A SAR (Synthetic Aperture Radar) sensor on board of a satellite returns observations of the time spent by a signal emitted by the sensor to cover the distance from the satellite to the Earth surface and back to the satellite sensor. Again this time is increased by the interaction with atmospheric water vapour.
From those observations, GNSS and SAR processing machineries procedures produce estimates of the total content of water vapour along the involved signal paths, expressed in terms of signal delay along the zenith direction (ZTD – Zenith Total Delay).
Differently from weather stations direct observations, which refer to the variable on the Earth surface, these products contain information on the meteorological variables along the signal paths. If properly ingested/assimilated by the NWP models they are expected to significantly improve the prediction of heavy rains.
To use these observations, assimilation techniques have been implemented in NWP models. Data assimilation is based on a sound mathematical theory and aims to find the best estimate of the atmospheric state by blending numerical model outputs and the observations themselves. The output of a data assimilation is called ‘analysis’ and it can be used as initial condition for a NWP model forecast. In case of ZTD observations, this process consists of a modification of the NWP variables in such a way that the resulting water vapour content matches as much as possible the corresponding observed values. Assimilating ZTD has already been shown to have a positive impact on heavy rains forecast.
One of the TWIGA challenges is to showcase how this augmented information can be used by NWP models in Africa by profitably deriving GNSS and SAR water vapour products from low-cost sensors and freely available satellite SAR images, respectively.
A low-cost GNSS network has been installed in Uganda and another one will be installed in Kenya. SAR images have been collected from the European Copernicus Sentinel 1 satellites. Water vapour products from those sources will be used for NWP model assimilation experiments.
KMD (Kenya) and SAWS (South Africa) meteorological agencies are directly involved in these experiments, thus gaining the expertise to be able to exploit the newly available water vapour products.
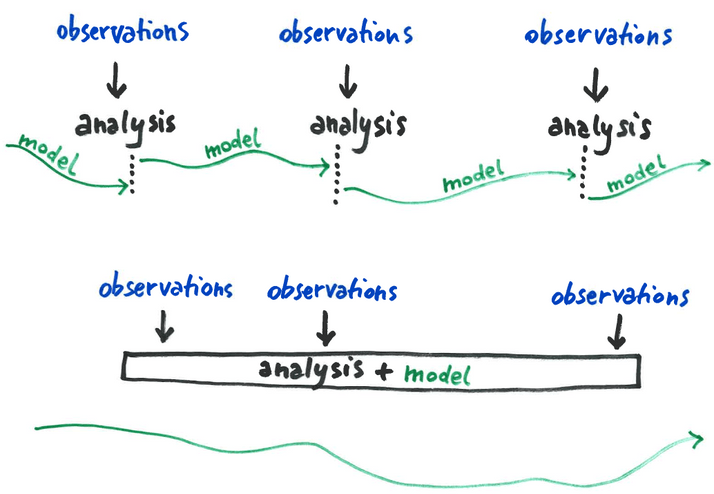
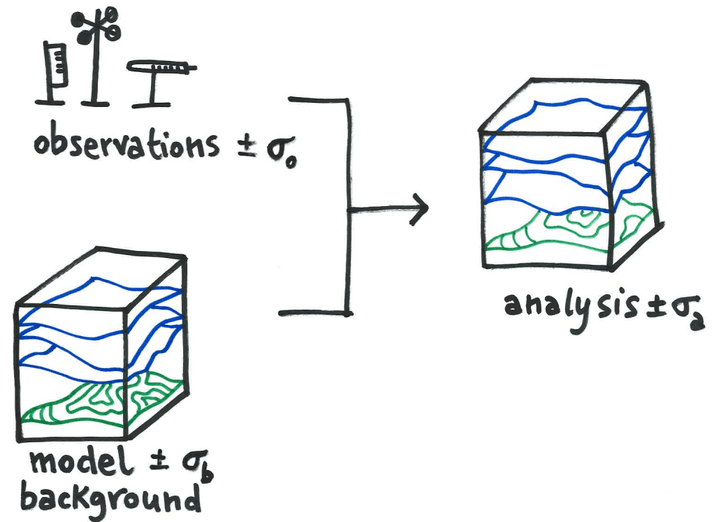
Edited by Giovanna Venuti and Agostino Meroni